Deepfake Detection Solutions for Effective Prevention of Deepfakes
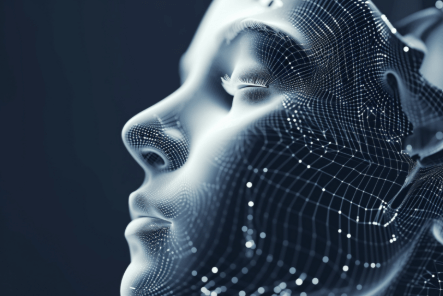
The technology has advanced to the point where manipulatively manufactured synthetic media of targeted individuals is spread via the internet for a variety of purposes. This fake media, also known as deepfakes, is developed with modern technology, making it difficult to distinguish between actual and fake identities. Even modern biometric identification technologies fail to detect fake identities because they appear so convincing and legitimate. Deepfake attacks are used by cybercriminals to propagate misleading information, promote fake adverts, exploit the victim’s social image, and alter people’s views. The majority of them are created to transmit sexual content, and porn deepfakes primarily target women. Artificial intelligence and machine learning are used to generate very sophisticated deepfakes, which blur the line between genuine and fake faces. Developing AI deepfake detection is critical for properly detecting and mitigating AI-generated deepfakes. Here are some indicators that can be used to identify the spoof:
- Inappropriate lighting and irregular shadow
- Unnatural movements
- Blurred edges
- Facial expressions anomalies
- Unmatched Lip-synching
- Robotic movements
How Deepfake Detection Works
There are numerous methods for detecting AI-generated deepfake videos. These can be identified by examining the visuals of the content. Some unusual motions in the video can assist in identifying the fake content. Furthermore, the margins of the face in a deepfake video are hazy and unclear. It can also be detected by closely observing the muscle movements while smiling and blinking. Also, voice patterns can help detect deepfake films. The AI-generated video cannot replicate some of the inherent variances of a real human.
Furthermore, tools such as machine learning and deep learning can help detect deepfakes. In machine learning, the system is trained by comparing fake and actual movies so that the system can distinguish between the two. Later in the process, the algorithm identifies even minor spoofs of AI-generated content. Additionally, deep learning can be employed for detection. It operates by utilizing a big database and samples. It is trained to interpret videos and images using existing datasets. This system can detect changes that are not visible to the human eye.
Advancements in Deepfake Detection Technology
AI protection systems must be constantly updated to include the most recent ways of deepfake generation. As a result, despite its seeming enormous promise, various challenges remain in the way of its general implementation.
As a result, collaborative AI models combined with rapidly evolving deepfake technologies are the future of deepfake detection. For example, these advanced systems would use speech recognition, image recognition, and behavior analysis to construct a comprehensive detection strategy; the issue has been developing such a system. Addressing issues like deep false variation across media types and attaining general success with cross-platform integration without cultural and language sensitivity are challenges that necessitate collaboration among platforms, governments, and AI experts.
Challenges Faced in the Detection Process
Deepfake technology evolves, and detection solutions evolve. The improved deepfake technology produces information that is nearly real and impossible for humans to identify. Some devices are unable to detect in hostile environments. However, sophisticated technologies are being developed to detect the spoof even under adverse and challenging settings. Furthermore, the methods are inefficient and require too much time for detection, which might lead to the rapid proliferation of fraudulent information. In addition, certain movies and images are detected as fraudulent even though they are genuine and legitimate. These technical flaws can also lead to the failure of the technology. The technology and its algorithms should be updated over time due to the increasing number of deepfakes. The advancement will lead to the efficient detection of spoofed content timely.
Conclusion
Technology evolution can spark innovation, but it should not violate privacy. Advancements and innovations are critical for progress, and they must not result in the misuse of anyone’s data. It is also critical to develop ethical norms and restrictions as the development progresses to avoid undesirable consequences. Furthermore, being vigilant can help avoid deepfakes; nonetheless, it is critical to use powerful deepfake detection online technologies that can detect fake identities in real-time. AI-powered deepfake detection solutions are highly accurate and can detect anomalies in seconds because they are trained on a large number of identities.